10th International Conference on
Evolutionary Multi-Criterion Optimization
March 10-13, 2019
East Lansing, Michigan, USA
In Partnership with HEEDS Design Space Exploration
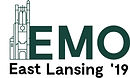
Tutorials
1) Evolutionary Many-Objective Optimization
by Hisao Ishibuchi
Chair Professor
Department of Computer Science and Engineering
Southern University of Science Technology (SUSTech), China

Abstract:
Recently, evolutionary many-objective optimization has been a hot research topic in the evolutionary multi-objective optimization (EMO) community. In this tutorial, first I briefly explain the popularity of evolutionary multi-objective and many-objective research using some statistics on publications and citations of related papers. Next I explain the following well-known difficulties in evolutionary many-objective optimization: (i) Pareto dominance does not work well in fitness evaluation, (ii) A huge number of solutions are needed to approximate the entire Pareto front, (iii) Visualization of obtained solutions is difficult, (vi) Selection of a single final solution is difficult, (v) Observation of search behavior of many-objective algorithms is difficult, and (vi) Usefulness of crossover is degraded. Especially, the first difficulty is explained in detail using experimental results on some test problems (e.g., many-objective knapsack problems, DTLZ, WFG, Minus-DTLZ, Minus-WFG) of three classes of EMO algorithms: Pareto dominance-based, indicator-based and decomposition-based algorithms. Then I explain difficulties in performance evaluations of evolutionary multi-objective and many-objective algorithms. The difficulties include the design of computational experiments (e.g., specification of the population size and the termination condition), the choice of test problems and the specification of performance indicators. Finally, I suggest some promising future research directions.
Short Bio
Hisao Ishibuchi received the BS and MS degrees from Kyoto University in 1985 and 1987, respectively. In 1992, he received the Ph. D. degree from Osaka Prefecture University where he was a professor since 1999. From April 2017, he is with Department of Computer Science and Engineering, Southern University of Science Technology (SUSTech), Shenzhen, China as a Chair Professor. He received Best Paper Awards from GECCO 2004, HIS-NCEI 2006, FUZZ-IEEE 2009, WAC 2010, SCIS & ISIS 2010, FUZZ-IEEE 2011, ACIIDS 2015 and GECCO 2017, 2018. He also received a 2007 JSPS (Japan Society for the Promotion of Science) Prize and a 2019 IEEE CIS Fuzzy Systems Pioneer Award. He was the IEEE CIS Vice-President for Technical Activities (2010-2013), an IEEE CIS Distinguished Lecturer (2015-2017), and the President of the Japan EC Society (2016-2018). Currently, he is the Editor-in-Chief of IEEE CI Magazine (2014-2019) and an IEEE CIS AdCom member (2014-2019). He is also an Associate Editor of IEEE TEVC, IEEE Access, and IEEE T-Cyb. He is an IEEE Fellow. In 2018, he was selected in the “Recruitment Program of Global Experts for Foreign Experts” known as the “Thousand Talents Program” in China.
2) Incorporating Preference Information in Multiobjective Optimization
by Kaisa Miettinen
Professor
University of Jyvaskyla, Industrial Optimization Group, Faculty of Information

Abstract:
Multiobjective optimization problems are a subset of multiple criteria decision making (MCDM) problems. In the presence of multiple conflicting objectives, we have so-called Pareto optimal solutions involving different trade-offs among the objectives. Typically, to be able to compare them, we need additional preference information from a decision maker, a person with domain expertise. By solving multiobjective optimization problems we mean finding a Pareto optimal solution that best meets the desires of a decision maker.
Multiobjective optimization methods can be classified based on the role of the decision maker in the solution process. We discuss different classes and methods as well as types of preference information they involve. We pay special attention at interactive methods, where the decision maker is actively involved in the solution process by iteratively providing preference information. In this way, (s)he can learn about the trade-offs, modify preferences if needed while learning, concentrate on promising solutions and gain confidence in the final solution. We also touch some ways of hybridizing elements of MCDM and evolutionary approaches
Short Bio
Kaisa Miettinen is Professor of Industrial Optimization of the University of Jyvaskyla. Her research interests include theory, methods, applications and software of nonlinear multiobjective optimization including interactive and evolutionary approaches. She heads the Research Group on Industrial Optimization and is the director of the thematic research area called Decision Analytics utilizing Causal Models and Multiobjective Optimization (DEMO). She has authored about 170 refereed journal, proceedings and collection papers, edited 14 proceedings, collections and special issues and written a monograph Nonlinear Multiobjective Optimization. She is a member of the Finnish Academy of Science and Letters, Section of Science and the Immediate-Past President of the International Society on Multiple Criteria Decision Making (MCDM). She belongs to the editorial boards of seven international journals and the Steering Committee of Evolutionary Multi-Criterion Optimization. She has previously worked at IIASA, International Institute for Applied Systems Analysis in Austria, KTH Royal Institute of Technology in Stockholm, Sweden and Helsinki School of Economics, Finland. In 2017, she received the Georg Cantor Award of the International Society on MCDM for independent inquiry in developing innovative ideas in the theory and methodology.